Date/Time
Date(s) - September 16, 2022
11:45 am - 12:35 pm
Location
Weil Hall 406
Categories No Categories
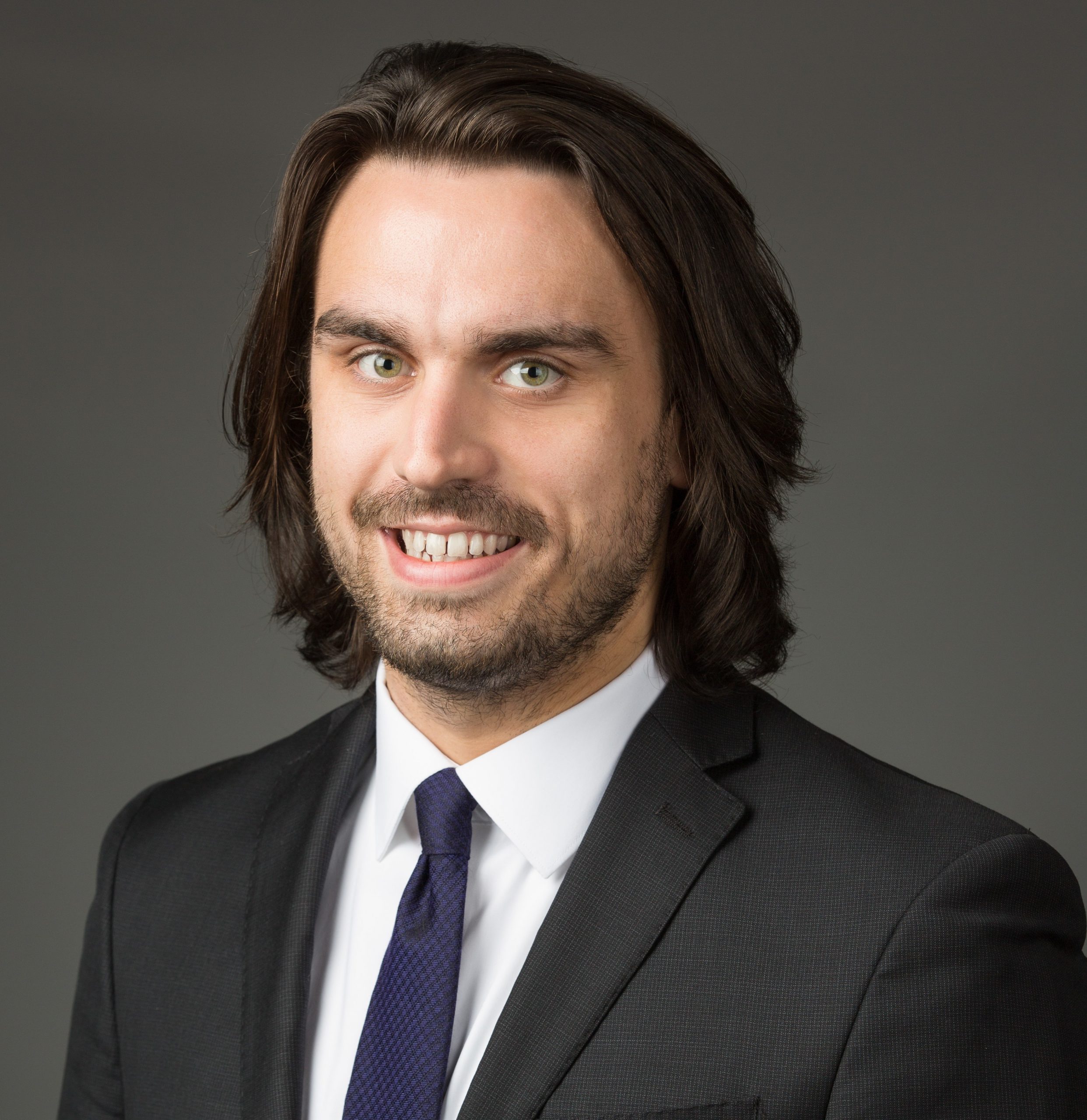
Matthew Hale
Date : Friday, September 16, 2022
Mode : In-Person
Affiliation: University of Florida
Bio: Visit Page
Title: Linear regularizers enforce the strict saddle property
Abstract: Non-convex optimization has grown rapidly in recent years, driven in part by the use of neural networks and other learning systems. The study of non-convex optimization problems is more complex than it is for convex problems, though the research community has recently identified properties of non-convex problems that allow them to be analyzed and solved effectively. One such property is the so-called “strict saddle property”, which is a form of topological non-degeneracy that ensures that optimization algorithms do not converge to saddle points. The strict-saddle property is often simply assumed to hold, though it can be difficult to actually verify for unstructured problems. Moreover, there do not exist methods to enforce the strict saddle property when it fails to hold inherently, and it is known to not hold for several important problems, including the training of neural networks with at least two hidden layers. This means that a wide range of practical problems cannot use a large body of theory that has been developed. To help close this gap, in this talk I will discuss recent work on regularizing a function with a linear term to enforce the strict saddle property. I will also provide justification for only regularizing locally, i.e., when the norm of the gradient falls below a certain threshold. This talk will analyze bifurcations that may result from this form of regularization, and then provide a selection rule for regularizers that depends only on the gradient of an objective function. This rule will be shown to guarantee that gradient descent will escape the neighborhoods around a broad class of non-strict saddle points. Along the way, I will demonstrate this behavior on common examples of non-strict saddle points, and I will provide numerical results from a well-known benchmark problem in the non-convex optimization literature.
Bio: Matthew Hale is an Assistant Professor of Mechanical and Aerospace Engineering at the University of Florida. He received his BSE in Electrical Engineering summa cum laude from the University of Pennsylvania in 2012, and his MS and PhD in Electrical and Computer Engineering from the Georgia Institute of Technology in 2015 and 2017, respectively. His research interests include multi-agent systems, mobile robotics, privacy in control, and distributed optimization. He received an NSF CAREER Award in 2020, an ONR YIP in 2022, and an Excellence Award for Assistant Professors at the University of Florida in 2022 (for being one of the 10 most outstanding assistant professors across all disciplines at the university).